Reduce Policy Acquisition Costs While Improving Customer Experience
Does your auto insurance underwriting process include the use of credit scores? If so, you know that the costs can really add up. You pay for reports for many prospects who never buy a policy. Furthermore, if you’re using credit reports late in the quoting process, you may be delivering a poor customer experience – a process some would describe as a bait and switch. Fortunately, there is an easy, affordable solution to these challenges.
Why Credit Scores Are Relevant
According to the FTC, the use of credit-based insurance scores has increased since the 1990s, largely because insurers found credit scores to be a strong predictor of risk. To test whether this correlation does indeed exist, the FTC conducted an analysis and found a consistent pattern of higher claims associated with individuals with lower credit-based insurance scores.
Credit scores can help insurers predict loss costs, so of course insurers want to use this information. The devil is in the details.
The Old Way: An Unintentional Bait and Switch
In a typical process, the driver completes a quick application to get a ballpark quote, which assumes a high credit score. If the driver likes the ballpark quote, they proceed with the purchase process and the credit report is pulled. Then, the insurer presents the final quote, which may be five times – or more – higher than the initial quote if the applicant has a low credit score.
From the insurer’s perspective, this process makes sense because they only pay for credit scores for serious applicants, thereby controlling policy acquisition costs.
From a driver’s perspective, it’s very frustrating if the second quote is very different from the initial quote. These drivers usually do not buy the policy and they may even leave a bad review.
Now the insurer has incurred the cost of the credit report, and their reputation has been damaged. If an agent is involved, the agent is also annoyed because they’ve wasted time and upset their client. They may avoid submitting business to the insurer in the future.
No one is happy with the way this process turned out.
It doesn’t tend to go the other way, either, with drivers being pleasantly surprised with a lower-than-expected rate at the second rate call. If the initial quote assumes a high credit score, the final rate is never less, strengthening the argument that the insurer engages in bait-and-switch tactics.
The Challenge: Create the Same Predictability with Other Variables
Credit scores provide valuable predictive information, but the old way of using credit scores in auto insurance underwriting leads to frustrated shoppers and high customer acquisition costs.
To solve these challenges, insurers need a different way of providing credit score predictability earlier in the process … without actually pulling the credit report.
Soteris In Action
To solve this challenge, we constructed a Credit Estimator model and trained our machine learning algorithm using insurance submissions with actual credit data attached. We then tested the model on another set of submissions that had not been used in training. This is the same way we evaluate performance of our algorithms for predicting loss ratio scores.
The actual credit scores were divided into 10 tiers. Our Credit Estimator model predicted the score within two tiers, roughly 80% of the time. Admittedly, it’s not perfect, but it does provide a much more accurate estimate than typical incumbent methods, such as just assuming all applicants are in the highest credit tier – and without pulling a credit score. For insurers who want to reduce auto insurance policy acquisition costs while protecting consumers from bait-and-switch frustrations, this is a game changing development.
The New Credit Estimator Process
With our Credit Estimator algorithm, insurers no longer have to assume a high credit score for all ballpark quotes.
- Insurers can use the Credit Estimator to get a credit score indicator early in the quoting process.
- This enables insurers to provide a more accurate ballpark quote based on an estimated score that is usually within one to two tiers of the actual score.
- If the driver is unhappy with the ballpark quote, they can back out right then, saving the insurer time and money and helping everyone avoid a lot of frustration.
- If the driver likes the ballpark quote, they can move forward, and the insurer can pull the actual credit score to finalize the premium.
The result is a more accurate and more affordable quoting process, without the bait and switch. The Credit Estimator is just one of the ways we’re applying machine learning to help insurers achieve greater rate accuracy.
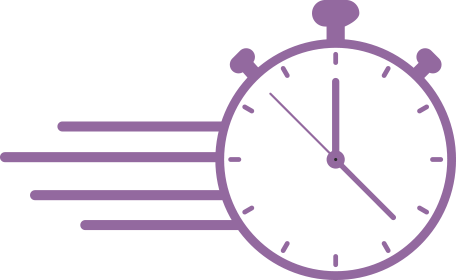