Overcoming Bias in Auto Insurance Underwriting
Bias in auto insurance underwriting is a serious risk. Biased practices can lead to lawsuits and regulatory action. They can also cause some drivers to miss out on good insurance rates, and some auto insurers to miss out on profitable business. Now, with the power of machine learning (ML), insurers can reduce the chance of biased risk selection by evaluating risks individually, instead of by segment.
How Does Bias Impact Auto Insurance Underwriting?
Auto insurers face dilemmas when deciding which underwriting factors to use for risk selection.
Historically, auto insurers have often relied on large-scale demographics as part of underwriting. Women pose less risk than men, so they pay less for coverage. Teenagers pose more risk than adults, so they pay more for coverage.
These generalizations have led to claims of bias. After all, even if it’s true that women as a whole have fewer claims than men as a whole, it’s not necessarily true that any individual woman will be a safer driver than any individual man. Charging different rates based on gender can be seen as a form of bias or even sex-based discrimination. In fact, Investopedia says that some states have banned gender-based auto insurance rating. Nevertheless, insurers in many states continue to use this factor because – historically – it’s been one of the few ways they can predict risk.
Things are starting to change now. The question is whether they’ll change for the better.
Concerns Over AI and Insurance Bias
As insurers adopt AI- and ML-powered strategies, some experts have raised concerns over whether this will increase bias. The worry is that algorithms tasked with determining insurance rates or approving claims may use discriminatory criteria to make decisions.
In some cases, this has even led to litigation. For example, according to LexisNexis, State Farm was sued over allegations that its AI tools discriminated against African American names.
However, AI does not necessarily have to lead to more bias. When applied appropriately, AI and machine learning could even help reduce bias. After all, humans tend to hold biases, and they are not always aware of their biases. By leveraging AI, human underwriters can possibly overcome their personal biases to adopt a more objective – and more accurate – approach to underwriting.
How to Solve the Problem of Bias
To avoid lawsuits and improve underwriting precision, auto insurers need to reduce bias in their underwriting. This is possible by evaluating the rate adequacy of individual drivers instead of large segments or classes of drivers.
ML can evaluate dozens of factors and how they intersect. Instead of accepting or rejecting applicants based on single factors, insurers can consider a larger number of factors linked to risk, such as population density, occupation, anti-theft features, and prior insurance. In the recent past, individual risk assessment presented an insurmountable challenge, but now, thanks to new ML capabilities, it’s possible to consider dozens of criteria and produce an individual rate adequacy score in less than one second. This advancement enables insurers to base decisions on individual risk scores, reducing the chance of bias against an entire segment or class.
Is it Time for a New Approach?
Soteris can revolutionize your auto insurance underwriting process with the power of machine learning. Instead of turning off entire segments, you can accurately evaluate an individual risk’s rate adequacy in less than one second.
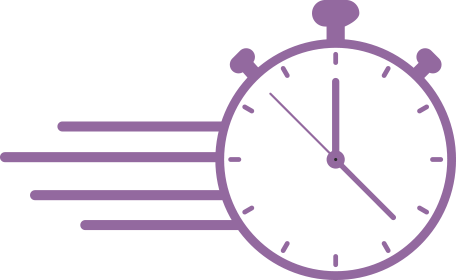