What Is Deep Learning and What Does It Mean For Insurance?
As the insurance industry delves deeper into AI, insurance professionals are encountering many new terms. However, many of these terms are not actually new. “Deep learning” is a good example. While the concept of deep learning is not new, recent applications and advances have brought it to the forefront.
Defining Deep Learning
If “deep learning” reminds you of the more common term “machine learning,” you’re on the right track. These two concepts are closely related.
- Machine learning refers to computer programs that are capable of learning without explicit instructions or with minimal human intervention by using algorithms.
- Deep learning is considered a subset or subfield of machine learning, and it is both more complex and more sophisticated.
As Amazon Web Services explains, deep learning uses more complex training methods, has a more complex neural network architecture and requires more data than many of the other subfields within machine learning. However, these differences don’t mean that deep learning is always superior. While deep learning is often more appropriate for uses involving unstructured data, other machine learning approaches are often well-suited for tasks involving structured data.
How Is Deep Learning Different from Artificial Intelligence?
Artificial intelligence, or AI, is an umbrella term that gets thrown around a lot to refer to a lot of different concepts. When people talk about artificial intelligence, they’re talking about computer programs that mimic human intelligence in some way. There are many different types and applications of AI, and deep learning is just one example. To make matters more complicated, it’s possible to break down AI in different ways, meaning that some of the categories may overlap.
For example, AI can be either weak or strong.
- Weak AI, often called artificial narrow intelligence, is capable of performing a specific task. However, while human intelligence is capable of performing a wide variety of tasks, artificial narrow intelligence is limited to one particular task.
- Strong AI, often called artificial general intelligence, reflects the kinds of general reasoning skills that we often associate with human intelligence. Like human intelligence, artificial general intelligence would be capable of performing a wide range of tasks – at least in theory. Artificial general intelligence has not yet been developed.
Here are some other AI-related terms you may have heard:
- Generative AI – a program that generates new content, such as images, text, video or audio. Generative AI is usually built upon deep learning algorithms.
- Large Language Models – Use deep learning algorithms to learn how to construct thoughts in languages from historical, human-generated content. Text-based generative AI programs are often driven by large language models.
- Computer vision – a program that can process and understand visual content, such as images and video. Sophisticated computer vision programs are usually trained using deep learning.
What Are the Applications of Deep Learning?
According to McKinsey & Company, deep learning isn’t new, but most people had never heard of it until ChatGPT was released. Like DALL-E and other generative AI tools, ChatGPT uses deep learning models to generate content based on prompts.
However, the applications of deep learning are not confined to generative AI. Many of the recent breakthroughs in AI are possible due to deep learning. According to Amazon Web Services, deep learning drives the technology found in many products, including digital assistants, fraud detection, automatic facial recognition systems and self-driving cars. Deep learning also drives computer vision and speech recognition capabilities, which allow programs to analyze images and sounds, respectively.
Deep learning capabilities have many applications in the insurance industry, from fraud detection to superior risk modeling. Deep learning can also be used in conjunction with other technologies to fully or partially automate underwriting and claims processes.
About Soteris
Soteris is revolutionizing auto insurance underwriting and risk selection. Our innovative approach begins with an analysis of your loss data and policy lifecycle activity, making it possible to rate risks on an individual policy basis at the point of sale – in less than one second.
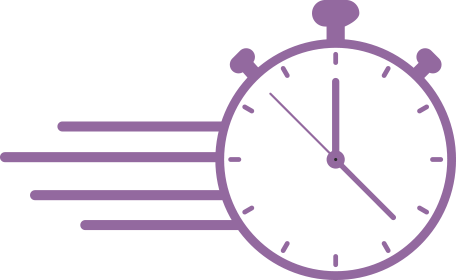